Activity
Ratings Progression
Challenge Categories
Challenges Entered
Identify user photos in the marketplace
Latest submissions
See Allfailed | 209364 | ||
failed | 209361 | ||
failed | 209358 |
Machine Learning for detection of early onset of Alzheimers
Latest submissions
See Allgraded | 140414 | ||
failed | 140408 | ||
graded | 140389 |
3D Seismic Image Interpretation by Machine Learning
Latest submissions
Multi-Agent Reinforcement Learning on Trains
Latest submissions
A benchmark for image-based food recognition
Latest submissions
See Allgraded | 115164 | ||
graded | 113600 | ||
graded | 113474 |
Latest submissions
Latest submissions
Predicting smell of molecular compounds
Latest submissions
See Allgraded | 91360 | ||
graded | 91359 | ||
graded | 91356 |
Classify images of snake species from around the world
Latest submissions
Latest submissions
See Allgraded | 107133 | ||
graded | 107131 | ||
graded | 107130 |
Latest submissions
See Allfailed | 108475 | ||
graded | 107464 | ||
graded | 107460 |
Grouping/Sorting players into their respective teams
Latest submissions
See Allgraded | 85577 | ||
graded | 85573 | ||
graded | 85571 |
Reinforcement Learning on Musculoskeletal Models
Latest submissions
See Allgraded | 12586 | ||
graded | 11549 | ||
graded | 11547 |
5 Problems 15 Days. Can you solve it all?
Latest submissions
Sample-efficient reinforcement learning in Minecraft
Latest submissions
Latest submissions
5 Problems 15 Days. Can you solve it all?
Latest submissions
See Allgraded | 64385 | ||
graded | 64384 | ||
ready | 64383 |
Help improve humanitarian crisis response through better NLP modeling
Latest submissions
See Allgraded | 58164 | ||
graded | 58163 | ||
graded | 58162 |
Immitation Learning for Autonomous Driving
Latest submissions
A new benchmark for Artificial Intelligence (AI) research in Reinforcement Learning
Latest submissions
5 PROBLEMS 3 WEEKS. CAN YOU SOLVE THEM ALL?
Latest submissions
Latest submissions
See Allgraded | 67780 | ||
graded | 67779 | ||
graded | 67777 |
Latest submissions
See Allgraded | 69090 |
Participant | Rating |
---|---|
![]() |
265 |
![]() |
136 |
![]() |
0 |
|
221 |
Participant | Rating |
---|---|
![]() |
265 |
|
221 |
-
rss_fete NeurIPS 2019: Learn to Move - Walk AroundView
-
rssfete Food Recognition ChallengeView
-
rssfete AMLD 2020 - Transfer Learning for International Crisis ResponseView
-
rssfete ORIENTMEView
-
rssfete AIcrowd Blitz - May 2020View
-
rssfete ECCV 2020 Commands 4 Autonomous VehiclesView
-
rssfete Hockey Team ClassificationView
-
rssfete Seismic Facies Identification ChallengeView
-
rssfete Learning to SmellView
-
rssfete Hockey: Player localizationView
-
rssfete Hockey Puck Tracking ChallengeView
-
rssfete Multi-Agent Behavior: Representation, Modeling, Measurement, and ApplicationsView
-
rssfete ADDI Alzheimers Detection ChallengeView
-
rssfete Visual Product Recognition Challenge 2023View
-
rssfete HackAPrompt 2023View
Food Recognition Challenge

Submission problem, can't start evaluation issue
Over 3 years agoI tried just now as well, and have the same issue @shivam

Train using mmdetection and submit via Colab (Round 2)!
About 4 years agoHey,
I think the baseline(food-round2) was created using this config file. You can compare this with the config you are using right now and try find out where the issue might be.

Cannot upload to the git even git lfs is checked ... windows using git tortoise
About 4 years agoNot unless it would be something compute intensive. Have you managed to get your code running locally using nvdia-docker, if your code works locally on nvidia-docker then maybe it is a server issue.

Step by step tutorials
About 4 years agoIβm not quite sure what errors you ran into while changing the model, Iβve made a notebook that does exactly that and makes a submission directly on colab. You can check it out here Train using mmdetection and submit via Colab!
Regards
Shraddhaa

Cannot upload to the git even git lfs is checked ... windows using git tortoise
About 4 years agoClearly for some reason the module has not been installed in your docker image. I would suggest adding RUN pip install scikit-image in your Dockerfile. Since this is a docker issue, I think you could find the problem by debugging locally. It is explained in the baseline repoβs readme.
It seems you have a submission in progress already, I hope youβve managed to solve your issue.

Train using mmdetection and submit via Colab (Round 2)!
About 4 years agoLEARN HOW TO SUBMIT | NO SSH | ON COLAB | DIRECTLY!!!
Hey everyone,
Since a lot of people seem to be finding it difficult to submit, weβve converted the baseline code using mmdetection into a colab notebook which allows you to submit directly via colab. If you are not using mmdetection you can still check this notebook out and have a look at the submission steps. If you have any issues or need any help do feel free to post here.
MMdetection Starter(DIRECT SUBMIT!)
Regards
AICrowd Team

Cannot upload to the git even git lfs is checked ... windows using git tortoise
About 4 years agoCan you check your .gitignore and see if .pth is mentioned there, if so remove it

Not able to ssh to gitlab
About 4 years agoCan you try running ssh -T git@gitlab.com after adding your generated key to your gitlab account ? Steps are here

New Starter Notebook + paperspace
About 4 years agoHey everyone,
We know that computing resources may be really difficult to come by, especially for beginners, so we have written a new starter notebook that allows you to train a MaskRCNN model directly on Colab.
This dataset and notebook correspond to the Food Recognition Challenge being held on AICrowd.
In this Notebook, we will first do an analysis of the Food Recognition Dataset and then use maskrcnn for training on the dataset.
The ChallengeΒΆ
- Given Images of Food, we are asked to provide Instance Segmentation over the images for the food items.
- The Training Data is provided in the COCO format, making it simpler to load with pre-available COCO data processors in popular libraries.
- The test set provided in the public dataset is similar to Validation set, but with no annotations.
- The test set after submission is much larger and contains private images upon which every submission is evaluated.
- Pariticipants have to submit their trained model along with trained weights. Immediately after the submission the AICrowd Grader picks up the submitted model and produces inference on the private test set using Cloud GPUs.
- This requires Users to structure their repositories and follow a provided paradigm for submission.
- The AICrowd AutoGrader picks up the Dockerfile provided with the repository, builds it and then mounts the tests folder in the container. Once inference is made, the final results are checked with the ground truth.
For more submission related information, please check the AIcrowd Challenge page and the starter kit.
The NotebookΒΆ
- Installation of MaskRCNN
- Using MatterPort MaskRCNN Library and Making local inference with it
- Local Evaluation Using Matterport MaskRCNN
A bonus section on other resources to read is also added!
Dataset DownloadΒΆ
Note: By downloading this data you are argeeing to the competition rules specified here
!wget -q https://s3.eu-central-1.wasabisys.com/aicrowd-public-datasets/myfoodrepo/round-2/train.tar.gz
!wget -q https://s3.eu-central-1.wasabisys.com/aicrowd-public-datasets/myfoodrepo/round-2/val.tar.gz
!mkdir data
!mkdir data/val
!mkdir data/train
!tar -xf train.tar.gz -C data/train
!tar -xf val.tar.gz -C data/val
InstallationΒΆ
#Directories present
import numpy as np # linear algebra
import pandas as pd # data processing, CSV file I/O (e.g. pd.read_csv)
import os
for dirname, _, filenames in os.walk('data/'):
print(dirname)
import warnings
warnings.filterwarnings("ignore")
pip install -q -U numpy==1.16.1
import os
import sys
import random
import math
import numpy as np
import cv2
import matplotlib.pyplot as plt
import json
from imgaug import augmenters as iaa
from tqdm import tqdm
import pandas as pd
import glob
!pip install -q tensorflow-gpu==1.13.1
import tensorflow as tf
tf.__version__
DATA_DIR = 'data'
# Directory to save logs and trained model
ROOT_DIR = 'working'
!git clone https://www.github.com/matterport/Mask_RCNN.git
os.chdir('Mask_RCNN')
!pip install -q -r requirements.txt
!python setup.py -q install
# Import Mask RCNN
sys.path.append(os.path.join('.', 'Mask_RCNN')) # To find local version of the library
from mrcnn.config import Config
from mrcnn import utils
import mrcnn.model as modellib
from mrcnn import visualize
from mrcnn.model import log
!pip uninstall pycocotools -y
!pip install -q git+https://github.com/waleedka/coco.git#subdirectory=PythonAPI
from mrcnn import utils
import numpy as np
from pycocotools.coco import COCO
from pycocotools.cocoeval import COCOeval
from pycocotools import mask as maskUtils
MaskRCNNΒΆ
To train MaskRCNN, two things we have to define FoodChallengeDataset
that implements the Dataset
class of MaskRCNN and FoodChallengeConfig
that implements the Config
class.
The FoodChallengeDataset
helps define certain functions that allow us to load the data.
The FoodChallengeConfig
gives the information like NUM_CLASSES
, BACKBONE
, etc.
class FoodChallengeDataset(utils.Dataset):
def load_dataset(self, dataset_dir, load_small=False, return_coco=True):
""" Loads dataset released for the AICrowd Food Challenge
Params:
- dataset_dir : root directory of the dataset (can point to the train/val folder)
- load_small : Boolean value which signals if the annotations for all the images need to be loaded into the memory,
or if only a small subset of the same should be loaded into memory
"""
self.load_small = load_small
if self.load_small:
annotation_path = os.path.join(dataset_dir, "annotation-small.json")
else:
annotation_path = os.path.join(dataset_dir, "annotations.json")
image_dir = os.path.join(dataset_dir, "images")
print("Annotation Path ", annotation_path)
print("Image Dir ", image_dir)
assert os.path.exists(annotation_path) and os.path.exists(image_dir)
self.coco = COCO(annotation_path)
self.image_dir = image_dir
# Load all classes (Only Building in this version)
classIds = self.coco.getCatIds()
# Load all images
image_ids = list(self.coco.imgs.keys())
# register classes
for _class_id in classIds:
self.add_class("crowdai-food-challenge", _class_id, self.coco.loadCats(_class_id)[0]["name"])
# Register Images
for _img_id in image_ids:
assert(os.path.exists(os.path.join(image_dir, self.coco.imgs[_img_id]['file_name'])))
self.add_image(
"crowdai-food-challenge", image_id=_img_id,
path=os.path.join(image_dir, self.coco.imgs[_img_id]['file_name']),
width=self.coco.imgs[_img_id]["width"],
height=self.coco.imgs[_img_id]["height"],
annotations=self.coco.loadAnns(self.coco.getAnnIds(
imgIds=[_img_id],
catIds=classIds,
iscrowd=None)))
if return_coco:
return self.coco
def load_mask(self, image_id):
""" Loads instance mask for a given image
This function converts mask from the coco format to a
a bitmap [height, width, instance]
Params:
- image_id : reference id for a given image
Returns:
masks : A bool array of shape [height, width, instances] with
one mask per instance
class_ids : a 1D array of classIds of the corresponding instance masks
(In this version of the challenge it will be of shape [instances] and always be filled with the class-id of the "Building" class.)
"""
image_info = self.image_info[image_id]
assert image_info["source"] == "crowdai-food-challenge"
instance_masks = []
class_ids = []
annotations = self.image_info[image_id]["annotations"]
# Build mask of shape [height, width, instance_count] and list
# of class IDs that correspond to each channel of the mask.
for annotation in annotations:
class_id = self.map_source_class_id(
"crowdai-food-challenge.{}".format(annotation['category_id']))
if class_id:
m = self.annToMask(annotation, image_info["height"],
image_info["width"])
# Some objects are so small that they're less than 1 pixel area
# and end up rounded out. Skip those objects.
if m.max() < 1:
continue
# Ignore the notion of "is_crowd" as specified in the coco format
# as we donot have the said annotation in the current version of the dataset
instance_masks.append(m)
class_ids.append(class_id)
# Pack instance masks into an array
if class_ids:
mask = np.stack(instance_masks, axis=2)
class_ids = np.array(class_ids, dtype=np.int32)
return mask, class_ids
else:
# Call super class to return an empty mask
return super(FoodChallengeDataset, self).load_mask(image_id)
def image_reference(self, image_id):
"""Return a reference for a particular image
Ideally you this function is supposed to return a URL
but in this case, we will simply return the image_id
"""
return "crowdai-food-challenge::{}".format(image_id)
# The following two functions are from pycocotools with a few changes.
def annToRLE(self, ann, height, width):
"""
Convert annotation which can be polygons, uncompressed RLE to RLE.
:return: binary mask (numpy 2D array)
"""
segm = ann['segmentation']
if isinstance(segm, list):
# polygon -- a single object might consist of multiple parts
# we merge all parts into one mask rle code
rles = maskUtils.frPyObjects(segm, height, width)
rle = maskUtils.merge(rles)
elif isinstance(segm['counts'], list):
# uncompressed RLE
rle = maskUtils.frPyObjects(segm, height, width)
else:
# rle
rle = ann['segmentation']
return rle
def annToMask(self, ann, height, width):
"""
Convert annotation which can be polygons, uncompressed RLE, or RLE to binary mask.
:return: binary mask (numpy 2D array)
"""
rle = self.annToRLE(ann, height, width)
m = maskUtils.decode(rle)
return m
class FoodChallengeConfig(Config):
"""Configuration for training on data in MS COCO format.
Derives from the base Config class and overrides values specific
to the COCO dataset.
"""
# Give the configuration a recognizable name
NAME = "crowdai-food-challenge"
# We use a GPU with 12GB memory, which can fit two images.
# Adjust down if you use a smaller GPU.
IMAGES_PER_GPU = 4
# Uncomment to train on 8 GPUs (default is 1)
GPU_COUNT = 1
BACKBONE = 'resnet50'
# Number of classes (including background)
NUM_CLASSES = 62 # 1 Background + 61 classes
STEPS_PER_EPOCH=150
VALIDATION_STEPS=50
LEARNING_RATE=0.001
IMAGE_MAX_DIM=256
IMAGE_MIN_DIM=256
config = FoodChallengeConfig()
config.display()
You can change other values in the FoodChallengeConfig
as well and try out different combinations for best results!
!mkdir pretrained
PRETRAINED_MODEL_PATH = os.path.join("pretrained", "mask_rcnn_coco.h5")
LOGS_DIRECTORY = os.path.join(ROOT_DIR, "logs")
if not os.path.exists(PRETRAINED_MODEL_PATH):
utils.download_trained_weights(PRETRAINED_MODEL_PATH)
from keras import backend as K
K.tensorflow_backend._get_available_gpus()
import keras.backend
K = keras.backend.backend()
if K=='tensorflow':
keras.backend.common.image_dim_ordering()
model = modellib.MaskRCNN(mode="training", config=config, model_dir=LOGS_DIRECTORY)
model_path = PRETRAINED_MODEL_PATH
model.load_weights(model_path, by_name=True, exclude=[
"mrcnn_class_logits", "mrcnn_bbox_fc",
"mrcnn_bbox", "mrcnn_mask"])
dataset_train = FoodChallengeDataset()
dataset_train.load_dataset('/content/data/train', load_small=False)
dataset_train.prepare()
dataset_val = FoodChallengeDataset()
val_coco = dataset_val.load_dataset(dataset_dir='/content/data/val', load_small=False, return_coco=True)
dataset_val.prepare()
class_names = dataset_train.class_names
# If you don't have the correct classes here, there must be some error in your DatasetConfig
assert len(class_names)==62, "Please check DatasetConfig"
class_names
Lets start training!!ΒΆ
print("Training network")
model.train(dataset_train, dataset_val,
learning_rate=config.LEARNING_RATE,
epochs=15,
layers='heads')
model_path = model.find_last()
model_path
class InferenceConfig(FoodChallengeConfig):
GPU_COUNT = 1
IMAGES_PER_GPU = 1
NUM_CLASSES = 62 # 1 Background + 61 classes
IMAGE_MAX_DIM=256
IMAGE_MIN_DIM=256
NAME = "food"
DETECTION_MIN_CONFIDENCE=0
inference_config = InferenceConfig()
inference_config.display()
# Recreate the model in inference mode
model = modellib.MaskRCNN(mode='inference',
config=inference_config,
model_dir=ROOT_DIR)
# Load trained weights (fill in path to trained weights here)
assert model_path != "", "Provide path to trained weights"
print("Loading weights from ", model_path)
model.load_weights(model_path, by_name=True)
# Show few example of ground truth vs. predictions on the validation dataset
dataset = dataset_val
fig = plt.figure(figsize=(10, 30))
for i in range(4):
image_id = random.choice(dataset.image_ids)
original_image, image_meta, gt_class_id, gt_bbox, gt_mask =\
modellib.load_image_gt(dataset_val, inference_config,
image_id, use_mini_mask=False)
print(original_image.shape)
plt.subplot(6, 2, 2*i + 1)
visualize.display_instances(original_image, gt_bbox, gt_mask, gt_class_id,
dataset.class_names, ax=fig.axes[-1])
plt.subplot(6, 2, 2*i + 2)
results = model.detect([original_image]) #, verbose=1)
r = results[0]
visualize.display_instances(original_image, r['rois'], r['masks'], r['class_ids'],
dataset.class_names, r['scores'], ax=fig.axes[-1])
import json
with open('/content/data/val/annotations.json') as json_file:
data = json.load(json_file)
d = {}
for x in data["categories"]:
d[x["name"]]=x["id"]
id_category = [0]
for x in dataset.class_names[1:]:
id_category.append(d[x])
#id_category
import tqdm
import skimage
files = glob.glob(os.path.join('/content/data/val/test_images/images', "*.jpg"))
_final_object = []
for file in tqdm.tqdm(files):
images = [skimage.io.imread(file) ]
#if(len(images)!= inference_config.IMAGES_PER_GPU):
# images = images + [images[-1]]*(inference_config.BATCH_SIZE - len(images))
predictions = model.detect(images, verbose=0)
#print(file)
for _idx, r in enumerate(predictions):
image_id = int(file.split("/")[-1].replace(".jpg",""))
for _idx, class_id in enumerate(r["class_ids"]):
if class_id > 0:
mask = r["masks"].astype(np.uint8)[:, :, _idx]
bbox = np.around(r["rois"][_idx], 1)
bbox = [float(x) for x in bbox]
_result = {}
_result["image_id"] = image_id
_result["category_id"] = id_category[class_id]
_result["score"] = float(r["scores"][_idx])
_mask = maskUtils.encode(np.asfortranarray(mask))
_mask["counts"] = _mask["counts"].decode("UTF-8")
_result["segmentation"] = _mask
_result["bbox"] = [bbox[1], bbox[0], bbox[3] - bbox[1], bbox[2] - bbox[0]]
_final_object.append(_result)
fp = open('/content/output.json', "w")
import json
print("Writing JSON...")
fp.write(json.dumps(_final_object))
fp.close()
submission_file = json.loads(open("/content/output.json").read())
len(submission_file)
type(submission_file)
import random
import json
import numpy as np
import argparse
import base64
import glob
import os
from PIL import Image
from pycocotools.coco import COCO
GROUND_TRUTH_ANNOTATION_PATH = "/content/data/val/annotations.json"
ground_truth_annotations = COCO(GROUND_TRUTH_ANNOTATION_PATH)
submission_file = json.loads(open("/content/output.json").read())
results = ground_truth_annotations.loadRes(submission_file)
cocoEval = COCOeval(ground_truth_annotations, results, 'segm')
cocoEval.evaluate()
cocoEval.accumulate()
cocoEval.summarize()
This, in addition to the existing Mask RCNN baseline repo should allow you to plug and play models for easy submission and experimentation.
As an alternative to Colab, Paperspace is an amazing option. Their gradient community notebooks let you use free cloud GPUβs and CPUβs and also provide internal storage that let you save models and resume training after the deployment time expires.
Regards
AICrowd Team

Cannot upload to the git even git lfs is checked ... windows using git tortoise
About 4 years agohave you setup git-lfs properly? These are the steps I used:
(check that git-lfs is setup correctly in your git configuration files)
git lfs install
track the model files, if the models are saved as model.pth for example, use the following to track all pth files
git lfs track '*.pth'
Next, you need to add .gitattributes
to your git repository.
git add .gitattributes
Now that this is all set up, you should be able add the model to the git repo by
git add .
git commit -m "commit message"
git push -u origin master
Let me know if this helped.
-Shraddhaa

Where to submit the repository link?
About 4 years agoTo submit youβll need to create a private git repository at https://gitlab.aicrowd.com with the contents of your submission(this is where all your files need to be,along with the appropriate directory structure mentioned in the starter-kit readme), and push a git tag corresponding to the version of your repository youβd like to submit.
The starter-kit has more instructions on how you can submit. Hope this helped.
Regards,
Shraddhaa
Learning to Smell

Where to start? 5 ways to learn 2 smell!
Over 3 years agoHi everyone!
@rohitmidha23 and me are undergrad students studying computer science, and found this challenge particularly interesting to explore the applications of ML in Chemistry. We have written a notebook that explores 5 ways to attempt this challenge. It includes baselines for
- ChemBERTa
- Graph Conv Networks
- MultiTaskClassifier using Molecular Fingerprints
- Sklearn Classifiers (Random Forest etc.) using Molecular Fingerprints
- Chemception (2D representation of molecules)
Check it out @ https://colab.research.google.com/drive/1-RedHEQSAVKUowOx2p-QoKthxayRshUa?usp=sharing
The most difficult task in this challenge is trying to get good representations of SMILES that is understandable for ML algorithms and we have tried to give examples on how that has been done in the past for these kind of tasks.
We hope that this notebook helps out other beginners like ourselves.
As always we are open to any feedback, suggestions and criticism!
If you found our work helpful, do drop us a !
Seismic Facies Identification Challenge

[Explainer]: A Noob Code-First Notebook
Over 3 years agoA Noob Code-First Notebook
The title is self-explanatory. Nothing too major, just some visualizations and a baseline model that we hope can help all those looking to start out.
Some really cool 3D visualizations for you to interact and play around with. Geologists in the community, looking to you to make more sense of the data and maybe even share some insights before the challenge ends .
Hereβs a sneak peak!
Check out the code here: https://colab.research.google.com/drive/1obka8aIo5zD4eJ96_FvCNdygNhXVUWOA#scrollTo=c9R9ZyYR9gCH
Hope this helps!

π Explained by the Community | Win 4 x DJI Mavic Drones
Over 3 years agoA Noob Code-First Notebook
The title is self-explanatory. Nothing too major, just some visualizations and a baseline model that we hope can help all those looking to start out.
Some really cool 3D visualizations for you to interact and play around with. Geologists in the community, looking to you to make more sense of the data and maybe even share some insights before the challenge ends .
Hereβs a sneak peak!
Check out the code here: https://colab.research.google.com/drive/1obka8aIo5zD4eJ96_FvCNdygNhXVUWOA#scrollTo=c9R9ZyYR9gCH
Hope this helps!
Hockey Team Classification

Request to see individual submission scores
Over 3 years agoHey @jason_brumwell,
Would it be possible to see the score of each submission? Currently, we can only see the submission that scored the highest on the leaderboard. This would really help understand how different approaches work for this task. Would this be possible? or is there a specific reason for not showing the scores?
Regards
Shraddhaa

About the evaluation metric
Over 3 years agoWould it be possible to know more about the evaluation metrics used for this challenge, like how the primary score and secondary score is calculated? @jason_brumwell
FOODC

Image ambiguity
Almost 4 years agoHi @jakub_bartczuk,
Thanks for your observations and feedback. The images in this dataset were collected from real users who track their daily food habits by taking pictures of food items they consume, and hence reflects the actual distribution of the data in the wild !
As you would expect there would be a few overlapping food items as well as some level of wrongly annotated data. Having gone through the data myself, there is a significant portion of images that can be classified as just one thing. There definitely are some exceptions as you have found. For example here is a visualization of all the images in the hard cheese class.
Some of the images have multiple food items, and hence as pointed out by you, there is significant merit in treating this as a multi-label classification problem. As a matter of fact, we plan to release a much larger version of this dataset with individual segmentations of all the different food items in each of the images, modelled as an image segmentation task. The said task would be a research challenge and not a part of the educational Blitz related initiatives. But we assure you, the nuanced data distributions and class imbalances will continue to be well represented even in the larger dataset : because it is what the real world distribution is.
At the same time, as mentioned in the forums a few times, we wanted to come up with a simplified classification problem from the original dataset as an easy-to-get-started problem for many of the community members.
We appreciate your inputs and the points you raised around the problem formulation, and are sure all of them would be well addressed when the larger dataset is released at the end of this month.
As this iteration of the AIcrowd Blitz ends, we hope we will be successful in aggregating all the activity that happened around these starter problems, and hope that we will be able to have continued engagement from community members like yourself even in the research challenges that we will organize as extensions to these problems.
Regards
Shraddhaa
ORIENTME

Colors in the Cube
Almost 4 years agoHey,
I do think the color is orange. You can check this discussion to see images of how the dataset was created.
AMLD 2020 - Transfer Learning for International...

Rssfete and tearth: Thank you so much
Over 4 years ago@student Do let us know if you are attending the conference, weβd love to see you there.
Congrats and good luck!
Notebooks
-
Where to start? 5 ways to learn 2 smell! We have written a notebook that explores 5 ways to attempt this challenge.shraddhaa_mohanΒ· Over 3 years ago
-
[Explainer]: A Noob Code-First Notebook Just some visualizations and a baseline model that we hope can help all those looking to start out.shraddhaa_mohanΒ· Over 3 years ago
MaskRCNN integrated with WandB and DIRECT SUBMIT FROM COLAB!
Over 3 years agoHi everyone!
@rohitmidha23 and me have been following this challenge for quite a while. We have written a starter notebook using MaskRCNN. We further integrate MaskRCNN with WandB which really helps to keep track of the various experiments that anyone might want to run. Check out our runs here
We also added functionality to view predictions of the model on the validation set as the model trains.
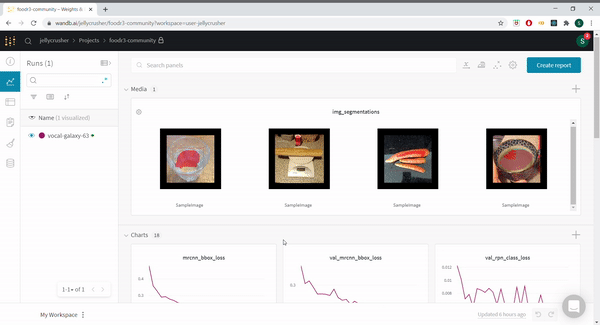
You can find the notebook @ https://colab.research.google.com/drive/1D8jC9GdHhCyoGB-8bJogW-lSO21MeTKF?usp=sharing
We trained the model on 40 classes alone due to Colabβs timing restrictions. However we provide a GitHub repo using which you can train on the entire dataset.
We also made a submission repo, using which you can submit models trained using the code above. The model we trained gets a precision score of 0.135 on the test set.
We hope that this notebook helps out other participants.
As always we are open to any feedback, suggestions and criticism!
If you found our work helpful, do drop us a
!